As Artificial Intelligence (AI) enters various facets of life, it is crucial to be aware of the potential risks that come with this powerful technology. AI security risks pose significant challenges and can have far-reaching consequences. They range from data breaches and privacy concerns to copyright infringement and vulnerabilities in the infrastructure. In this article, we explore these risks as well as the strategies to mitigate them effectively.
1. Data Breach and Privacy Risks
One of the most prominent AI security risks is the potential for data breaches and privacy violations. As AI systems rely heavily on large datasets to train their algorithms and make predictions, this puts sensitive information at risk.
Generally, the risk of cybersecurity breaches has grown over the years.
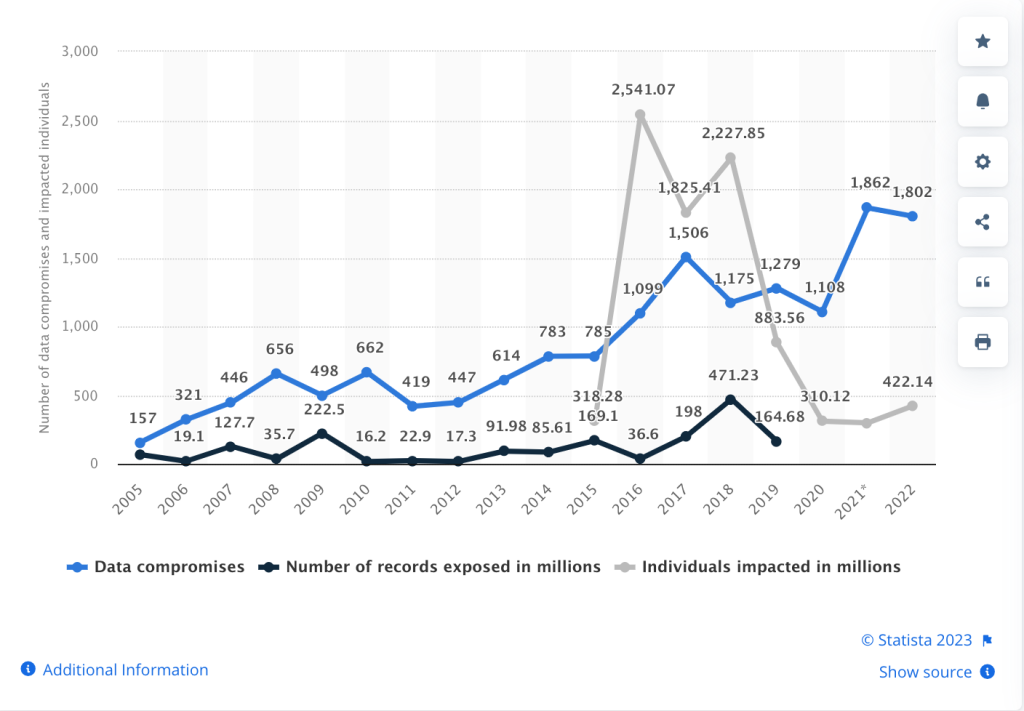
When it comes to data breaches, the consequences can be far-reaching and devastating. Not only can personal information be exposed, but financial data, medical records, and even trade secrets can be compromised. The aftermath of a data breach can be costly, both financially and in terms of reputation. Companies may face legal consequences, loss of customer trust, and damage to their brand image.
“According to a 2019 Forrester Research report, 80% of cybersecurity decision-makers expected AI to increase the scale and speed of attacks, and 66% expected AI “to conduct attacks that no human could conceive of.” (CSO Online, “Emerging Cyberthreats,” Sept 2023.)
Financial institutions, in particular, are attractive targets of cyberattacks due to the valuable information they possess. With AI systems being increasingly used in the financial sector, the risks associated with data breaches are amplified.
Healthcare organizations also face significant risks when it comes to data breaches. Patient records and medical data are becoming prime targets for hackers. A breach in a healthcare system could not only compromise the privacy of patients but also have life-threatening consequences. For example, if a hacker gains access to a hospital’s AI-powered diagnostic system and manipulates the data, incorrect diagnoses and treatments could occur, putting patients’ lives at risk.
Prevent Data Breaches with Effective Tools
To combat these risks, organizations need to implement robust security measures. This includes encryption of sensitive data, regular security audits, and employee training on cybersecurity best practices. Additionally, AI systems themselves need to be designed with security in mind. This involves building in safeguards to protect against unauthorized access and ensuring that data is stored and transmitted securely.
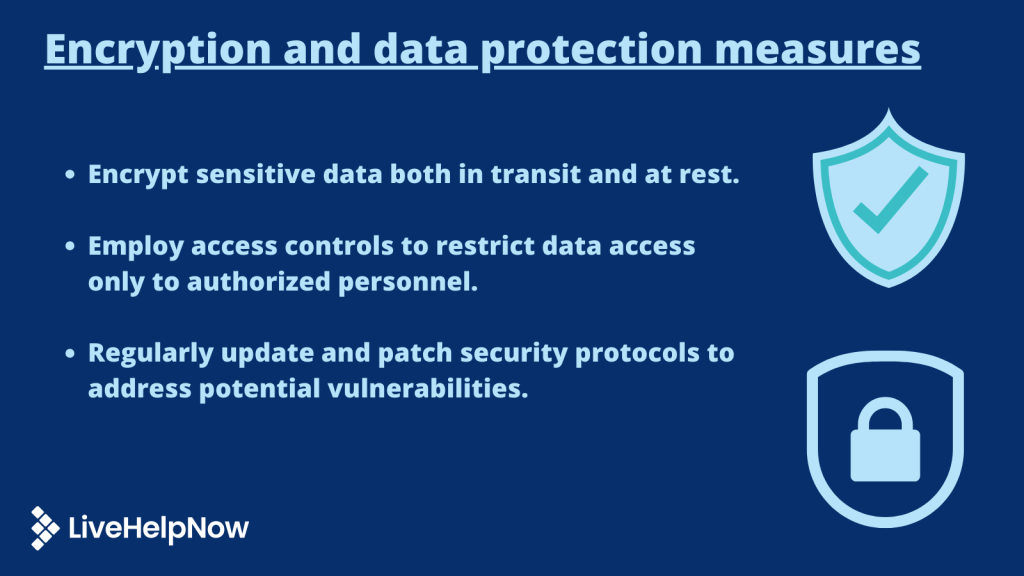
Privacy regulations such as HIPAA and RFPA must remain in focus as companies embrace AI technology in their operations. These regulations impose strict requirements on organizations, including the need to obtain explicit consent for data collection and to provide individuals with the right to access and delete their data. Failure to comply with these regulations can result in significant fines and legal consequences.
2. Model Poisoning
Another significant AI security risk is model poisoning. In this attack, malicious actors manipulate the training data used to develop AI models, resulting in biased or misleading outcomes. Model poisoning is a sophisticated technique that poses a serious threat to the integrity and reliability of AI systems. It involves injecting malicious data into the training dataset, which can lead to the AI model learning incorrect patterns or making biased decisions. This can have severe implications, particularly in critical applications such as healthcare diagnostics or autonomous vehicles. The consequences of such attacks can be devastating, as they can compromise the safety and effectiveness of AI-powered systems.
The recent emergence of a tool called Nightshade serves as an example of model poisoning. In this case, the tool serves artists who wish to protect their work against AI, so the poisoning occurs with human interests in focus. However, the example shows model poisoning at work.
“A new tool lets artists add invisible changes to the pixels in their art before they upload it online so that if it’s scraped into an AI training set, it can cause the resulting model to break in chaotic and unpredictable ways. The tool, called Nightshade, is intended as a way to fight back against AI companies that use artists’ work to train their models without the creator’s permission. Using it to “poison” this training data could damage future iterations of image-generating AI models” (MIT Technology Review, “New data poisoning tool lets artists fight back against generative AI,” Oct. 2023)
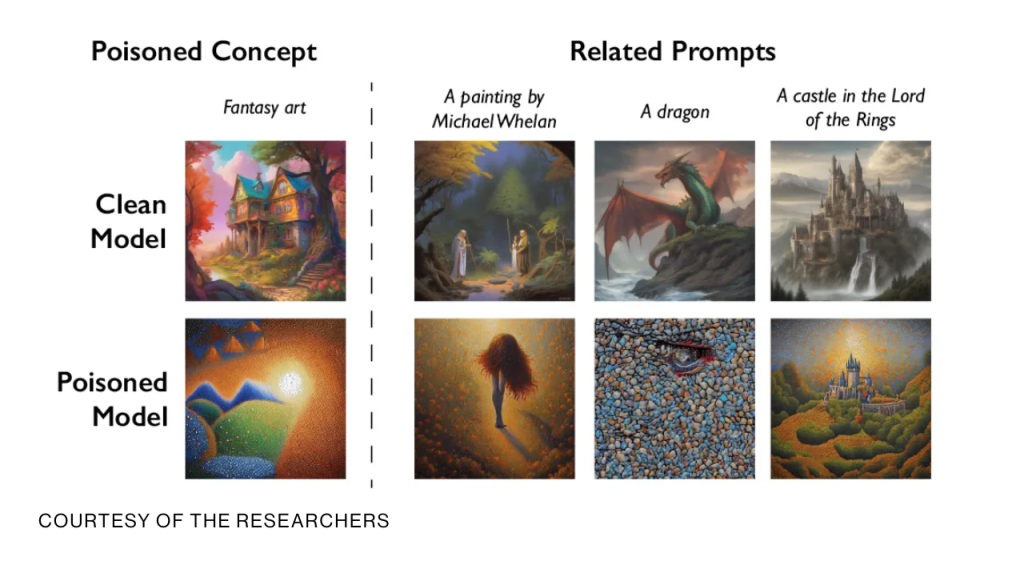
Defences Against AI Model Poisoning
Ensuring the security and trustworthiness of AI models is crucial, especially in domains where human lives are at stake. The potential for model poisoning attacks to manipulate AI systems and compromise their decision-making capabilities is a cause for concern. Should a company elect to use AI technologies in its operations, it’s imperative to invest in robust defense mechanisms and techniques to detect and mitigate model poisoning attacks.
One approach to mitigating model poisoning attacks is to implement rigorous data validation processes during the training phase. This involves carefully examining the training dataset for any signs of tampering or malicious injections. Additionally, organizations can employ anomaly detection algorithms to identify any unusual patterns or biases in the AI model’s predictions.
Continuous monitoring of AI models is also essential to detect any signs of model poisoning after deployment. Regular audits and evaluations can help identify any deviations from expected behavior and prompt further investigation. Moreover, collaboration between AI researchers, cybersecurity experts, and domain specialists is crucial to stay ahead of emerging threats and develop effective countermeasures.
3. Copyright Infringement and Plagiarism
With the increasing use of AI in content creation, copyright infringement, and plagiarism have become significant concerns. AI-powered tools can generate original content, imitating the style and tone of human authors, leading to a rise in automated plagiarism.
“The bot doesn’t work perfectly. It has a tendency to “hallucinate” facts that aren’t strictly true, which technology analyst Benedict Evans described as “like an undergraduate confidently answering a question for which it didn’t attend any lectures. It looks like a confident bullshitter that can write very convincing nonsense.” (Scott Aaronson, OpenAI researcher, The Guardian, Sept. 2022)
AI algorithms are designed to analyze vast amounts of data and generate content based on patterns and existing works. While this can be incredibly useful in speeding up content production, it also poses significant AI security risks.
The Need for Policy and Regulations to Combat “AIgiarism”
With AI continuously learning and improving, the need for stricter measures to protect intellectual property rights skyrockets. To address AI security concerns that come with a threat of “AIgiarism,” or AI-assisted plagiarism, organizations must implement robust systems to verify the authenticity and originality of AI-generated content.
This includes conducting thorough checks to ensure that the content produced does not infringe upon existing copyrights. Additionally, clear guidelines and policies should be established to govern the use of AI tools and to educate content creators about the ethical implications of plagiarism.
Furthermore, it is crucial for organizations to foster a culture of integrity and respect for intellectual property rights. This involves promoting awareness and understanding of copyright laws and encouraging employees to uphold ethical standards in their work. By instilling a sense of responsibility and accountability, organizations can minimize the risk of copyright infringement and plagiarism.
4. Vulnerabilities in AI Infrastructure
Like any other technology, AI can also be vulnerable to attacks on its underlying infrastructure. This includes the hardware, software, and networks that support AI systems. Exploiting these vulnerabilities can lead to unauthorized access, data manipulation, or even complete system compromise.
Adversarial attacks exploit vulnerabilities in AI models by subtly manipulating input data, leading to misclassifications or incorrect predictions. By introducing carefully crafted perturbations, attackers can deceive image recognition or natural language processing systems, compromising the reliability and integrity of AI-driven decision-making processes.
“Any impairment in a critical infrastructure function, any missed opportunity to invest in infrastructure remediation or resilience creates an exploitation pathway for a potential adversary to either hold infrastructure segments hostage or to actually actively exploit them at a time and place of their choosing,” warned David Mussington, executive assistant director for infrastructure security at CISA, the Cybersecurity and Infrastructure Security Agency.” (Report from AFCEA Bethesda’s Energy, Infrastructure, and Environment Summit 2023.)
4 Ways Safeguard Critical Infrastructure
- Investing in Resilience: Fortify critical infrastructure against potential threats.
- Leveraging AI: Artificial intelligence can help stay ahead of adversaries by rapidly identifying and mitigating cyber threats.
- Government Collaboration: The federal government should work closely with private sector partners and continue cross-pollination among agencies.
- Funding and Prioritization: Critical infrastructure security initiatives are essential as the White House’s recent executive order requires actionable plans with measurable results.
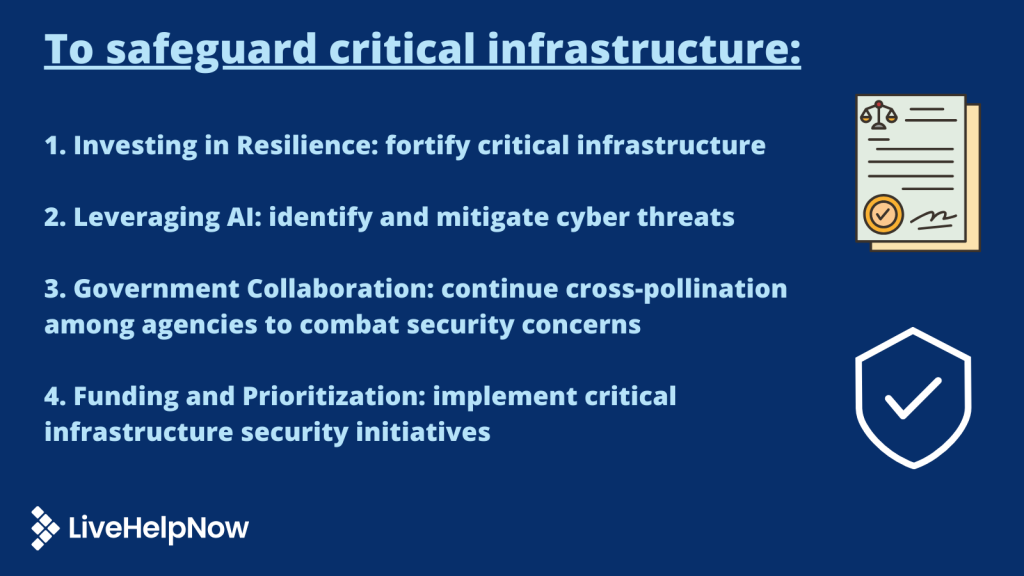
5. Model Inversion
Model inversion is a privacy-focused attack on AI systems where adversaries attempt to extract sensitive information about the training data or the model itself. In this attack, attackers leverage the output of the AI model to infer details about the input data used during training. By repeatedly querying the model with inputs and analyzing the corresponding outputs, they seek to reverse-engineer aspects of the original data or uncover patterns in the model’s learning process.
This attack poses a significant threat to confidentiality and privacy, especially in applications where the underlying data is sensitive, such as in medical diagnostics or personal identification systems. Model inversion can reveal details about individuals present in the training data, potentially leading to unintended disclosure of personal information.
Enhancing Data to Defend Against Model Inversion
Defending against model inversion involves enhancing the privacy of the training data, employing differential privacy techniques, and implementing robustness measures within the AI model. By mitigating the risk of model inversion, organizations can better safeguard sensitive information and ensure the ethical and secure deployment of AI technologies in various domains.
Here are several strategies to mitigate the risk of model inversion attacks:
- Differential Privacy: Integrate differential privacy techniques during the training process. This adds noise to the training data, making it more challenging for attackers to extract sensitive information while preserving the overall utility of the model.
- Limiting Output Information: Restrict the level of detail provided in model outputs, especially when dealing with sensitive data. Limiting the granularity of information disclosed helps reduce the potential for adversaries to infer details about the training data.
- Input Perturbation: Apply input perturbation techniques to training data. By introducing random variations or noise to the input data during the training phase, the model becomes less susceptible to reverse engineering, making it more challenging for attackers to infer specific details.
- Adversarial Training: Incorporate adversarial training methods during the model development process. Exposing the model to potential inversion attacks during training can enhance its robustness and resilience against such privacy breaches.
- Data Aggregation and Aggregation Techniques: Aggregate data at a higher level before using it for model training. This helps in obscuring fine-grained details and makes it more difficult for attackers to infer specifics about individual data points.
Keeping Pace with Evolving AI Security Challenges
As AI technology continues to evolve rapidly, security practices must keep pace to address new and emerging risks. Traditional security measures are often insufficient to protect against AI attacks. Therefore, organizations need to invest in specialized AI security solutions, such as anomaly detection algorithms or AI-driven threat intelligence platforms.
Additionally, collaboration within the industry is crucial to develop robust security frameworks. Sharing knowledge and best practices can help organizations stay ahead of malicious actors and create a united front against AI security risks.
Protecting Data from AI Security Risks
Safeguarding data from AI security threats requires a holistic approach that encompasses both technological and procedural measures. Encryption, access controls, and strict data governance policies are essential to protect sensitive information used in AI systems.
Mitigating Risks Through Effective Implementation
Effective implementation is key to mitigating AI security risks. Organizations must adopt a risk-based approach where security considerations are integrated from the inception of AI projects. This involves conducting thorough risk assessments, ensuring secure coding practices, and robustly testing the AI systems for vulnerabilities.
Furthermore, establishing an AI security incident response plan is essential to limit the impact of a potential breach. This plan should outline steps to contain the attack, recover compromised data, and minimize disruption to business operations.
As you consider the importance of mitigating AI security risks, remember that safeguarding your customer support systems is crucial. LiveHelpNow offers an omnichannel customer support suite that integrates seamlessly with AI to enhance security and efficiency. Our platform allows you to automate routine inquiries, freeing up your agents to tackle more complex issues and improve overall service quality. Embrace the transformative potential of AI with LiveHelpNow and experience a streamlined, productive customer service environment. Take the first step towards a more secure and efficient operation with a Free 30 Day Trial today.